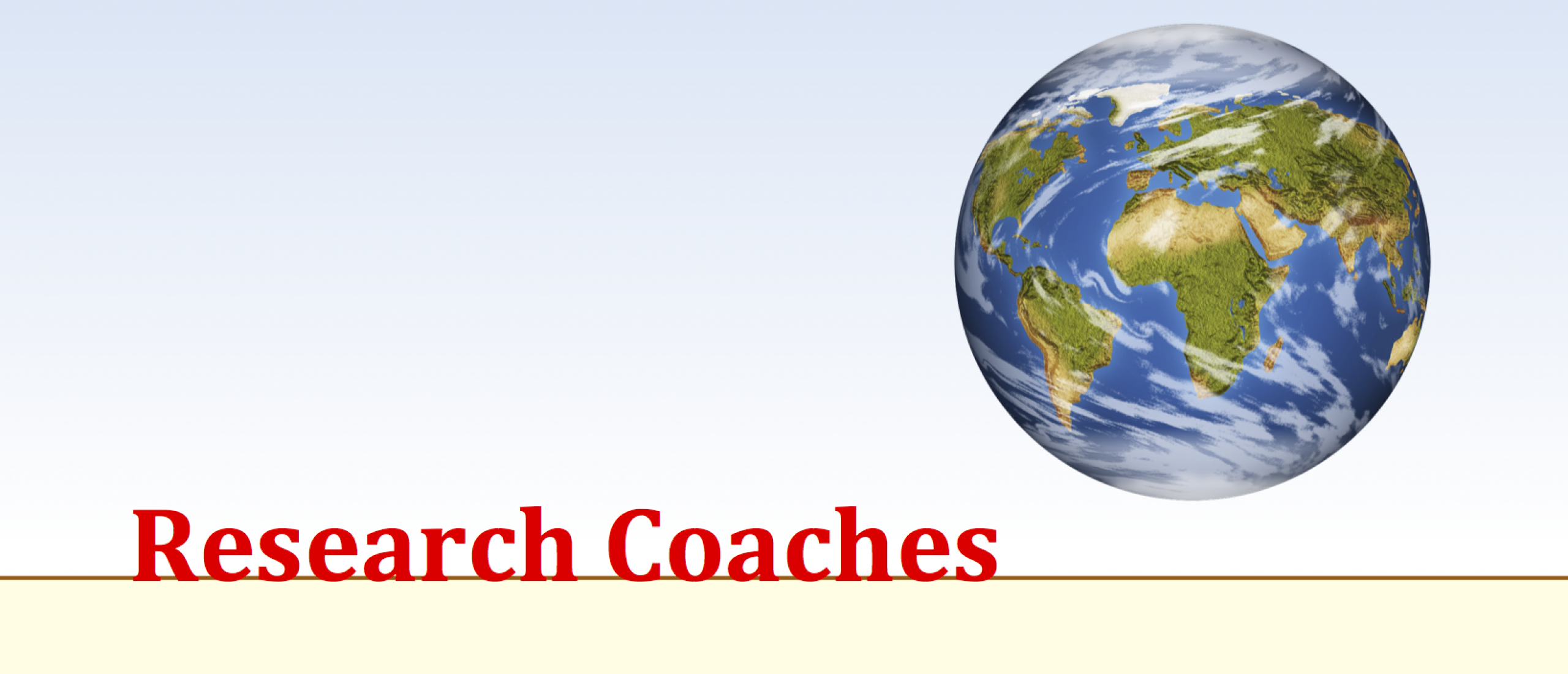
Multicollinearity
Multicollinearity is due to a high correlation between two or more predictive variables. The first indicator is the correlation coefficient between the variables. In addition in regression the VIF score can be computed.
If you want to predict a score, the correlation coefficient between two variables has to be computed. A low correlation coefficient indicates a low relationship between the variables. The higher the correlation coefficient the better the prediction is. If the correlation coefficient is 1, it means both variables measure exactly the same thing. For instance, in countries where women aren’t allowed to work, the income of the man is the same as the family income (with an extra condition that there are no children who contribute to the family income).
In the case of regression, the outcome must be predicted from one or more variables. For instance, the size of the house can be predicted out of family income and family size. Now, if one or more of the predictive variables have a high correlation, one of these is redundant. It means they measure the same thing and if one of these variables is deleted, it will not have any effect on the predicted outcome.
It may be clear that the correlation coefficient is highly indicative. When two or predictive variables correlate more than 0.80 in a regression analysis, one of the variables can be omitted. Best of all, keep the one that correlates the highest with the dependent variable (the to-predict-variable) in the model and leave the variable with the lowest correlation with the dependent variable out. Now the model will have the best predictors.
Though most of the time the correlation coefficient between predictive variables is a good indicator, sometimes a combination of variables leads to multicollinearity. To identify these combinations the Variable Inflation Factor (VIF) is computed.
I do not know how this value is computed. However, if only one predictive variable is used, the VIF value is 1. If variables are added, the value increases. Small increases indicate that the model is okay, however, how high should the VIF value become to indicate the model shows multicollinearity? Most authors in textbooks write that VIF values lower than 4 show that the model is okay. Some authors draw the line for the VIF values at 2. I think this value is too low. But you also have authors who retain variables in the model with VIF values up to 10. Best of all, the model is good for VIF values up to 4. Just follow the middle.
Use our SPSS-tutorials to analyse your data. It will go much faster.