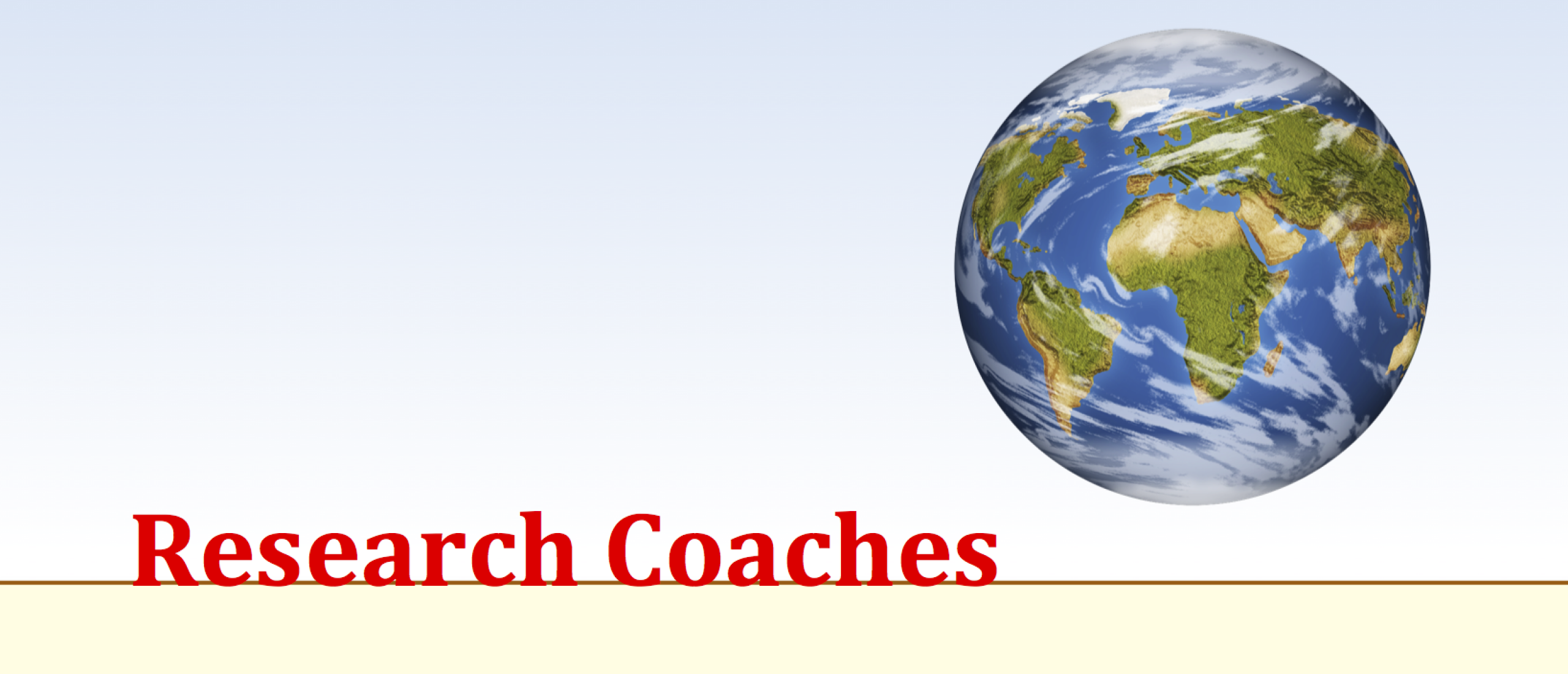
Moderation
Moderation is a theoretical issue assuming that a combination of aspects will have an impact on top of both aspects separately. This can be tested with regression analysis.
To test a moderation effect, first try to find out what you really want to prove. The combination of two aspects might have a positive effect, a negative effect or no effect at all. Most people always expect a positive effect in the regression analysis, and they are surprised when a negative effect occurs. Keep in mind that regression analysis is a technique to estimate an outcome, so the combination of two aspects may give a correction for overestimating or underestimating.
This example can clarify the effect of moderation. Children with a better concentration will show better results on an achievement test. Also, children who are motivated will show better results on an achievement test. And the combination of both makes them even better.
This theory can be tested. The children do some tests. They have to fill in a questionnaire about concentration, about motivation and finally they do an achievement test in for instance arithmetic. Between all aspects correlation coefficients can be computed, but it is better to perform a serial test with regression analysis. These equations can be tested:
y = a + b1 * x1
y = a + b2 * x2
y = a + b1 * x1 + b2 * x2
y = a + b1 * x1 + b2 * x2 + b3 * (x1 * x2)
Actually we are only interested in equation 4, because that is the one with the moderation effect. The others are used for explanation. In equation 1 the arithmetic score (y) is predicted merely from the score on the concentration test (x1). In equation 2 the arithmetic score is merely predicted based on the score on the motivation test (x2). In equation 3 the arithmetic score is predicted based on the score on the concentration test and the motivation test. And finally in equation 4 the combination of motivation and concentration is tested. As you can see, the combination is tested as the product of both sores and is has a regression coefficient (b3) of its own.
How to compute the interaction effect (x1 * x2)
The combination of concentration and motivation is called the interaction effect. The way it is presented here and elsewhere, looks like a simple product of the two variables. This however leads to a high score on collinearity, indicating that the individual effect of concentration and motivation has to be omitted from the equation.
To avoid this problem both variables have to be centred. This can be done in two ways.
The first one is probably the easiest: standardize all variables so their means are 0 and the standard deviations are 1. Then the interaction effect can be computed as the product of these standardised variables.
The second way to centre the variables is by computing the means and then computing the interaction effect: (x1 – mean(x1)) * (x2 – mean(x2)). Both ways will make a new variable and this one can be added to your regression analysis.
Interpreting the result of the moderation effect
If there is an moderation effect the regression coefficient (b3) should be statistically significant. If it’s not statistically significant it means there is no moderation effect: the combination of concentration times motivation doesn’t have a combined influence.
If the regression coefficient is statistically significant, then look at the sign. If the regression coefficient is positive, the change in the prediction is upwards. The score on the performance test is underestimated based on only the scores on concentration and motivation. If the regression coefficient is negative, the score on the performance test is overestimated based on the additive model where the scores for concentration and motivation are added. So there is a downward adjustment in the forecast.
With this final outcome the answer on your research question can be answered. Do not solely take notice of the significance of the regression coefficients (that is what most people do), but also take notice of the sign.