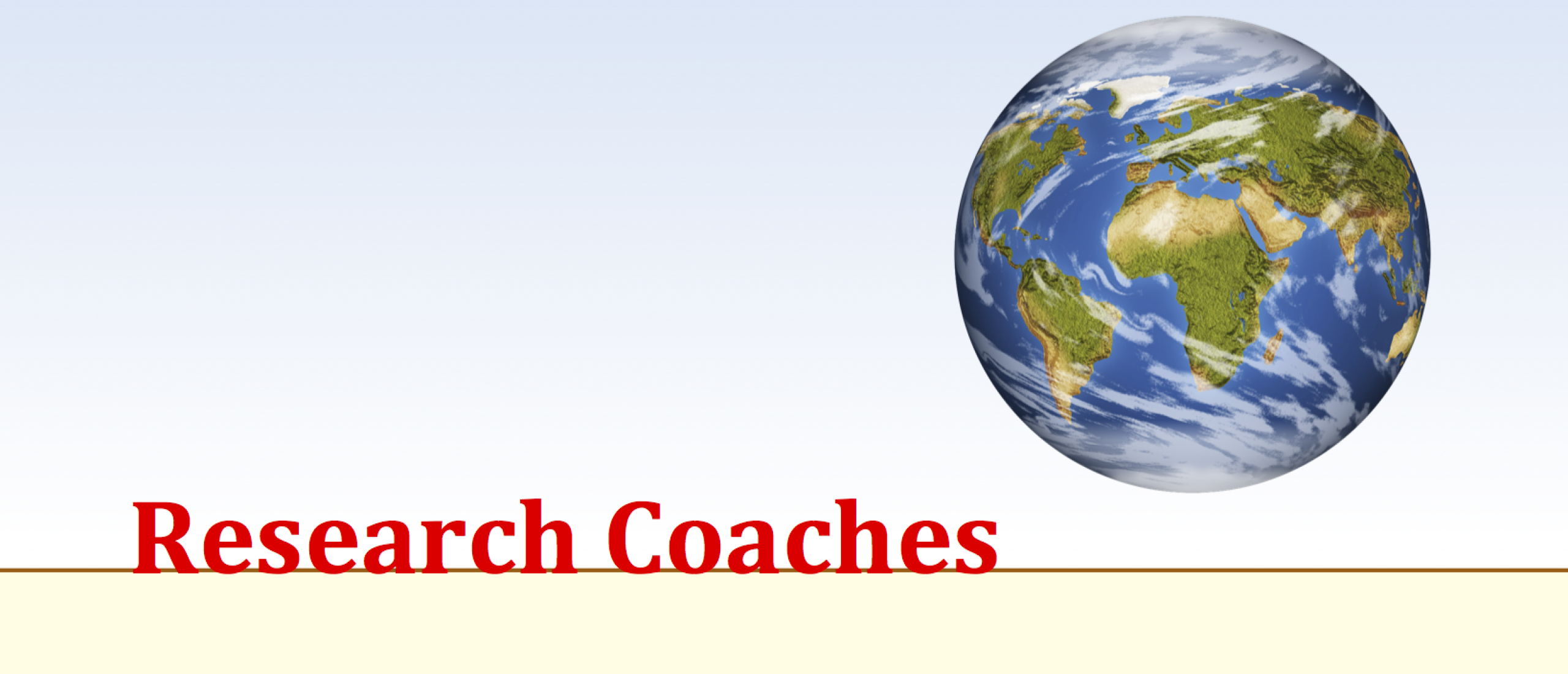
Outlier
An outlier is an extreme answer or a respondent who gives extreme answers that far are far removed from the given answers of the other respondents.
First of all notice that in this definition the answer is extreme and not the respondent. And it can be one answer or a whole bunch of answers, but it never means the respondent is extreme.
Second, notice that it’s about the answers compared to answers of the other respondents. This raises the question: what is extreme?
Extreme can be defined as the distance to the mean. A value that is far from the mean is an outlier, at least if it is really far away compared to the answers of the other respondents. The distance depends on the type of distribution. The dispersion in a distribution is determined by calculating the standard deviation. And now you can define an outlier: an outlier is a score that is more than 3 standard deviations away from the mean.
Is this a proper definition? No, it is not. If there are a lot of respondents and the answers are fairly normally distributed around the mean, by this definition 0.5% of the upper and 0.5% of the lower bound are said to be extreme while they are completely normal. And if you get rid of these respondents and start looking for outliers again, you will find new outliers that weren’t there before. If you keep on doing this you will end up with respondents close to the middle. And with only respondents close to the middle, the variable doesn’t discriminate enough. This makes the variable useless.
A few decades ago, values were considered as extreme if they were more than five standard deviations away from the mean. Now this may seem more fair, it is still an arbitrary decision.
It can even be a good decision not to remove outliers at all, because this is the reality. And do you want to change the reality because it fits better in your statistics? Yes, sometimes it is worthwhile because an outlier might give a total different view on the subject of your study. So sometimes it is better to remove an outlier. But not always.
What to do with outliers?
First of all, look at the data. Print it as a histogram and see if there are one or a few scores far away from the others. Then ask yourself if this is a possible answer. Maybe you or the respondent himself made a mistake in typing in the answer. Check it out and if this is the cause, then correct the answer and hopefully your outlier isn’t an outlier anymore.
Secondly, if the answer is correct but still extreme it doesn’t always influence the statistical outcome. Especially in large samples, sometimes the influence of an outlier is hardly noticed. How can you find out what the influence of an outlier is? Quite simple: do the analysis once with and once without the outlier. Compare both outcomes and ask yourself if you would draw a different conclusion. If so, the outlier has a big influence, if not the outlier has hardly any influence.
Should all answers of the respondent be removed?
Very often researchers remove all answers of the respondent who gave an extreme answer. Again, it is not the respondent who is extreme, it is usually just a few answers or scores. In my opinion it is not necessary to remove the respondent from the data. There is no doubt that all his answers are incorrect. Besides that, if you remove him it will weaken your research because it might influence the representativity or the statistical outcome due to a loss in the degrees of freedom. Read more about these topics on these pages.
Related topics to outliers:
Use our SPSS-tutorials to analyse your data. It will go much faster and easier.